Program Poker Ai
Computer scientists from the University of Alberta in Canada have programmed an AI poker player that can never lose across a series of hands of heads-up limit Texas hold'em.
Poker console game introduction. This tutorial is a reflection of what I had to program for a school’s mandatory project. I developed a simple C Poker console game with adjusted rules and logic. We wanted the Smart AI to play based on features and patterns, and not based on a set of rules, which is what the Dumb AI was doing. Once we created a Smart AI model, we started training it using Q-Learning. We used the Dumb AI's neural network weights to begin with, but after 10,000 epochs of training, stopped, due to extremely clear errors.
Named Cepheus, the program uses a strategy for a two-player game of heads-up limit Texas hold’em poker that’s so brilliant, statistical analysis says that even if a person spends their entire lifetime playing poker against this program, the program will never lose. The AI will only ever come out on top, or break even. It will never make a mistake, even not knowing what cards its opponent is holding.
And it’s not about winning every hand - it will get dealt dud cards as often as the next guy - but the AI has figured out how to turn even the worst situations around to come out on top. “[It] will lose if it's dealt an inferior hand, but it will minimise its losses as best as is mathematically possible and will slowly but surely take your money by making the 'perfect' decision in any given scenario,” says Jason Koebler at Motherboard. 'Heads-up limit hold'em, it can be said, has been ‘solved’.'
The difference between ‘limit’ and ‘no limit’ poker comes down to money - you’re either limited to set amounts you can bet, or you can bet as much as you like. The latter would be impossible for a computer program to solve, because predicting random and limitless amounts of money is a feat in and of itself. But that doesn’t make what this program can do any less impressive.
Compare what it’s doing to other games that have been ‘solved’ by AI players. Even our feeble human brains can figure out how to be unbeatable in naughts and crosses. Games like chess and checkers take the complexity way up a notch because of all the different possibilities at every turn, but all the information is still there on the board. The opponent can’t hide anything, other than their strategy, but that doesn’t matter, because the AI already knows every possible play, and has already figured out the perfect strategy for countering each move before the game has even started.
But what about poker? The program would be cheating if it knew what was on the two hidden cards its opponent holds in every round. As Koebler notes, Cepheus must somehow know how to navigate the 3 x 10^14 possible decisions in a game of limit poker, in which, at any given moment, not all the information is known to it. The University of Alberta team refers to this kind of game, where not all information is known, as an ‘imperfect-information’ game.
“The solutions for imperfect-information games require computers to handle the additional complication of not knowing exactly what the game’s state is, such as not knowing an opponent’s hand,” one of the team, Neil Burch, told Jeremy Hsu at IEEE Spectrum. “Such techniques require more computer memory and computing power.”
How much memory, exactly? Roughly 262 terabytes. Yikes. And what does it do with all that memory? Cepheus runs an algorithm called CFR+, which was invented by the team as an enhancement of an existing algorithm known as the counterfactual regret minimisation (CFR).
Regret minimisation is, essentially, about learning from your mistakes. So, as Arielle Duhaime-Ross explains at The Verge, if Cepheus thinks about the possibility of raising a bet, and decides to play randomly instead and loses, it will retrace its steps, figure out how much it could have won if it had raised, and will store that amount as a ‘regret value’.
This value is placed on every possible opportunity that this same decision is available to the computer, so it will avoid making the same mistake. This is very different from the way humans play - if we take a big loss, we focus on trying to win that back, rather than on how to use that information to perfect the rest of our game. Cepheus will continue to update itself with these regret values until it reaches what the team calls “perfect play”.
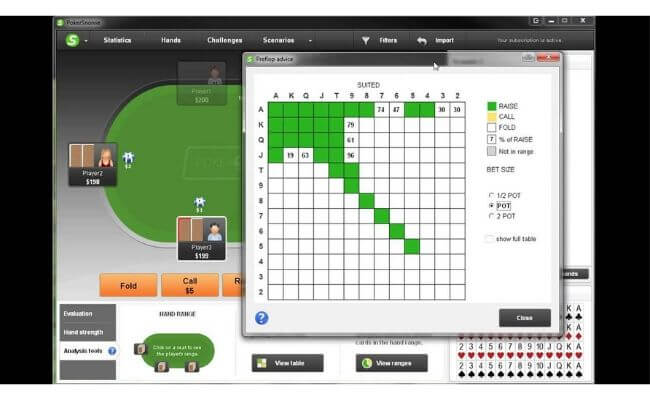
'CFR+ still works like the old CFR algorithms by gradually developing better solutions through playing thousands and hundreds of thousands of hands of poker,” Hsu writes at IEEE Spectrum. 'But it can develop a very good solution much faster than any past CFR algorithm by being more efficient; basically the equivalent of taking fewer, bigger steps toward the best solution.”
The team published their findings in the journal Science.
According to IEEE Spectrum, once the team had figured out the strategy, they managed to whittle the memory requirements down to less than 11 terabytes to store the counterfactual values, and 6 terabytes to compute the main strategy. It ended up taking 24 trillion hands of poker over 70 days, and 200 computers running the CFR+ algorithm with 32 GB of RAM and 24 central processing units to train Cepheus to ’solve’ the game. 'We could continue to train it, and it would continue to get better,” one of the team, Michael Bowlin, told Arielle Duhaime-Ross at The Verge. 'But we stopped at this point because we can’t tell it apart from being perfect.”
The team is now going to work on adjusting the algorithm to play heads-up no limit poker. They know, because of the variables, it will probably be impossible to create an ‘unbeatable’ AI player, but they hope to create a player that can beat the best human players in the world. That’s the goal. And they’re also thinking about how to use the technology to help governments and private companies create better security systems that can’t be hacked.
Want to try your hand against Cepheus? Click here to play against it. I just hope you enjoy the feeling of losing.
Sources: Motherboard, The Verge, IEEE Spectrum
Expert-Level Artificial Intelligence in Heads-Up No-Limit Poker
Links
Twitch YouTube Twitter
Downloads & Videos Media Contact
DeepStack bridges the gap between AI techniques for games of perfect information—like checkers, chess and Go—with ones for imperfect information games–like poker–to reason while it plays using “intuition” honed through deep learning to reassess its strategy with each decision.
With a study completed in December 2016 and published in Science in March 2017, DeepStack became the first AI capable of beating professional poker players at heads-up no-limit Texas hold'em poker.
DeepStack computes a strategy based on the current state of the game for only the remainder of the hand, not maintaining one for the full game, which leads to lower overall exploitability.
DeepStack avoids reasoning about the full remaining game by substituting computation beyond a certain depth with a fast-approximate estimate. Automatically trained with deep learning, DeepStack's “intuition” gives a gut feeling of the value of holding any cards in any situation.
DeepStack considers a reduced number of actions, allowing it to play at conventional human speeds. The system re-solves games in under five seconds using a simple gaming laptop with an Nvidia GPU.

/cdn.vox-cdn.com/uploads/chorus_image/image/64710190/957381514.jpg.0.jpg)
The first computer program to outplay human professionals at heads-up no-limit Hold'em poker
In a study completed December 2016 and involving 44,000 hands of poker, DeepStack defeated 11 professional poker players with only one outside the margin of statistical significance. Over all games played, DeepStack won 49 big blinds/100 (always folding would only lose 75 bb/100), over four standard deviations from zero, making it the first computer program to beat professional poker players in heads-up no-limit Texas hold'em poker.
Games are serious business
Don’t let the name fool you, “games” of imperfect information provide a general mathematical model that describes how decision-makers interact. AI research has a long history of using parlour games to study these models, but attention has been focused primarily on perfect information games, like checkers, chess or go. Poker is the quintessential game of imperfect information, where you and your opponent hold information that each other doesn't have (your cards).
Until now, competitive AI approaches in imperfect information games have typically reasoned about the entire game, producing a complete strategy prior to play. However, to make this approach feasible in heads-up no-limit Texas hold’em—a game with vastly more unique situations than there are atoms in the universe—a simplified abstraction of the game is often needed.
A fundamentally different approach
DeepStack is the first theoretically sound application of heuristic search methods—which have been famously successful in games like checkers, chess, and Go—to imperfect information games.
At the heart of DeepStack is continual re-solving, a sound local strategy computation that only considers situations as they arise during play. This lets DeepStack avoid computing a complete strategy in advance, skirting the need for explicit abstraction.
During re-solving, DeepStack doesn’t need to reason about the entire remainder of the game because it substitutes computation beyond a certain depth with a fast approximate estimate, DeepStack’s 'intuition' – a gut feeling of the value of holding any possible private cards in any possible poker situation.
Finally, DeepStack’s intuition, much like human intuition, needs to be trained. We train it with deep learning using examples generated from random poker situations.
DeepStack is theoretically sound, produces strategies substantially more difficult to exploit than abstraction-based techniques and defeats professional poker players at heads-up no-limit poker with statistical significance.
Download
Paper & Supplements
Hand Histories
Members (Front-back)
Michael Bowling, Dustin Morrill, Nolan Bard, Trevor Davis, Kevin Waugh, Michael Johanson, Viliam Lisý, Martin Schmid, Matej Moravčík, Neil Burch
low-variance Evaluation
The performance of DeepStack and its opponents was evaluated using AIVAT, a provably unbiased low-variance technique based on carefully constructed control variates. Thanks to this technique, which gives an unbiased performance estimate with 85% reduction in standard deviation, we can show statistical significance in matches with as few as 3,000 games.
Abstraction-based Approaches
Despite using ideas from abstraction, DeepStack is fundamentally different from abstraction-based approaches, which compute and store a strategy prior to play. While DeepStack restricts the number of actions in its lookahead trees, it has no need for explicit abstraction as each re-solve starts from the actual public state, meaning DeepStack always perfectly understands the current situation.
Professional Matches
We evaluated DeepStack by playing it against a pool of professional poker players recruited by the International Federation of Poker. 44,852 games were played by 33 players from 17 countries. Eleven players completed the requested 3,000 games with DeepStack beating all but one by a statistically-significant margin. Over all games played, DeepStack outperformed players by over four standard deviations from zero.
Heuristic Search
Program Poker Ai Play
At a conceptual level, DeepStack’s continual re-solving, “intuitive” local search and sparse lookahead trees describe heuristic search, which is responsible for many AI successes in perfect information games. Until DeepStack, no theoretically sound application of heuristic search was known in imperfect information games.